MVC Distance¶
See the tutorial for a description of Modified Velocity Centroids (MVC).
The distance metric for MVC is based on the t-statistics of the difference between the power spectrum slopes:
\(\beta_i\) and \(\sigma_{\beta_i}\) is the index and index uncertainty, respectively.
More information on the distance metric definitions can be found in Koch et al. 2017.
Using¶
The data in this tutorial are available here.
We need to import the MVC_Distance
class, along with a few other common packages:
>>> from turbustat.statistics import MVC_Distance
>>> from astropy.io import fits
>>> import matplotlib.pyplot as plt
MVC is the only (current) statistic in TurbuStat that requires multiple moment arrays. Because of this, the input for MVC_Distance
has a different format than the other distance metrics: a dictionary that contains the array and headers:
>>> moment0 = fits.open("Design4_flatrho_0021_00_radmc_moment0.fits")[0] # doctest: +SKIP
>>> centroid = fits.open("Design4_flatrho_0021_00_radmc_centroid.fits")[0] # doctest: +SKIP
>>> lwidth = fits.open("Design4_flatrho_0021_00_radmc_linewidth.fits")[0] # doctest: +SKIP
>>> data = {"moment0": [moment0.data, moment0.header],
... "centroid": [centroid.data, centroid.header],
... "linewidth": [lwidth.data, lwidth.header]} # doctest: +SKIP
And we create a second dictionary for the data set to compare with:
>>> moment0_fid = fits.open("Fiducial0_flatrho_0021_00_radmc_moment0.fits")[0] # doctest: +SKIP
>>> centroid_fid = fits.open("Fiducial0_flatrho_0021_00_radmc_centroid.fits")[0] # doctest: +SKIP
>>> lwidth_fid = fits.open("Fiducial0_flatrho_0021_00_radmc_linewidth.fits")[0] # doctest: +SKIP
>>> data_fid = {"moment0": [moment0.data, moment0.header],
... "centroid": [centroid.data, centroid.header],
... "linewidth": [lwidth.data, lwidth.header]} # doctest: +SKIP
These dictionaries can optionally include uncertainty arrays for the moments using the same format with keywords moment0_error
, centroid_error
, and linewidth_error
.
These dictionaries get passed to MVC_Distance
:
>>> mvc = MVC_Distance(data_fid, data) # doctest: +SKIP
To calculate the distance between the MVC power-spectra is calculated with:
>>> mvc.distance_metric(verbose=True, xunit=u.pix**-1) # doctest: +SKIP
OLS Regression Results
==============================================================================
Dep. Variable: y R-squared: 0.925
Model: OLS Adj. R-squared: 0.924
Method: Least Squares F-statistic: 378.5
Date: Tue, 13 Nov 2018 Prob (F-statistic): 8.18e-34
Time: 10:21:40 Log-Likelihood: -62.343
No. Observations: 91 AIC: 128.7
Df Residuals: 89 BIC: 133.7
Df Model: 1
Covariance Type: HC3
==============================================================================
coef std err z P>|z| [0.025 0.975]
------------------------------------------------------------------------------
const 15.2461 0.161 94.965 0.000 14.931 15.561
x1 -4.8788 0.251 -19.455 0.000 -5.370 -4.387
==============================================================================
Omnibus: 5.193 Durbin-Watson: 0.068
Prob(Omnibus): 0.075 Jarque-Bera (JB): 4.522
Skew: -0.459 Prob(JB): 0.104
Kurtosis: 2.408 Cond. No. 4.40
==============================================================================
OLS Regression Results
==============================================================================
Dep. Variable: y R-squared: 0.941
Model: OLS Adj. R-squared: 0.941
Method: Least Squares F-statistic: 477.5
Date: Tue, 13 Nov 2018 Prob (F-statistic): 1.55e-37
Time: 10:21:40 Log-Likelihood: -52.867
No. Observations: 91 AIC: 109.7
Df Residuals: 89 BIC: 114.8
Df Model: 1
Covariance Type: HC3
==============================================================================
coef std err z P>|z| [0.025 0.975]
------------------------------------------------------------------------------
const 14.0302 0.144 97.714 0.000 13.749 14.312
x1 -5.0144 0.229 -21.853 0.000 -5.464 -4.565
==============================================================================
Omnibus: 3.541 Durbin-Watson: 0.129
Prob(Omnibus): 0.170 Jarque-Bera (JB): 3.488
Skew: -0.469 Prob(JB): 0.175
Kurtosis: 2.800 Cond. No. 4.40
==============================================================================
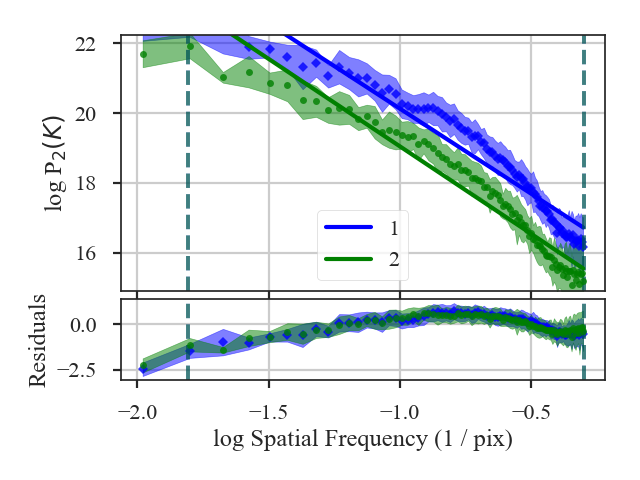
The MVC spectra are plotted in the figure and the fit summaries are printed out. The distance between the indices is:
>>> mvc.distance # doctest: +SKIP
0.3988169606167437
This is an awful fit. We want to limit where the spectra are fit. Keywords for MVC
can be passed with low_cut
, high_cut
, breaks
, pspec_kwargs
and pspec2_kwargs
. If separate parameters need to be set, a two-element list or array can be given to low_cut
, high_cut
and breaks
; the second element will be used for the second data set. For example, limiting the fit region can be done with:
>>> mvc = MVC_Distance(data_fid, data, low_cut=0.02 / u.pix,
... high_cut=0.4 / u.pix) # doctest: +SKIP
>>> mvc.distance_metric(verbose=True, xunit=u.pix**-1) # doctest: +SKIP
OLS Regression Results
==============================================================================
Dep. Variable: y R-squared: 0.946
Model: OLS Adj. R-squared: 0.942
Method: Least Squares F-statistic: 135.6
Date: Tue, 13 Nov 2018 Prob (F-statistic): 2.99e-08
Time: 10:36:41 Log-Likelihood: 10.700
No. Observations: 15 AIC: -17.40
Df Residuals: 13 BIC: -15.98
Df Model: 1
Covariance Type: HC3
==============================================================================
coef std err z P>|z| [0.025 0.975]
------------------------------------------------------------------------------
const 17.9988 0.266 67.588 0.000 17.477 18.521
x1 -2.5502 0.219 -11.647 0.000 -2.979 -2.121
==============================================================================
Omnibus: 1.189 Durbin-Watson: 2.376
Prob(Omnibus): 0.552 Jarque-Bera (JB): 0.814
Skew: -0.200 Prob(JB): 0.666
Kurtosis: 1.931 Cond. No. 13.5
==============================================================================
OLS Regression Results
==============================================================================
Dep. Variable: y R-squared: 0.951
Model: OLS Adj. R-squared: 0.948
Method: Least Squares F-statistic: 70.08
Date: Tue, 13 Nov 2018 Prob (F-statistic): 1.36e-06
Time: 10:36:41 Log-Likelihood: 10.420
No. Observations: 15 AIC: -16.84
Df Residuals: 13 BIC: -15.42
Df Model: 1
Covariance Type: HC3
==============================================================================
coef std err z P>|z| [0.025 0.975]
------------------------------------------------------------------------------
const 16.7135 0.390 42.879 0.000 15.950 17.477
x1 -2.7335 0.327 -8.371 0.000 -3.373 -2.094
==============================================================================
Omnibus: 0.831 Durbin-Watson: 2.076
Prob(Omnibus): 0.660 Jarque-Bera (JB): 0.621
Skew: -0.449 Prob(JB): 0.733
Kurtosis: 2.568 Cond. No. 13.5
==============================================================================
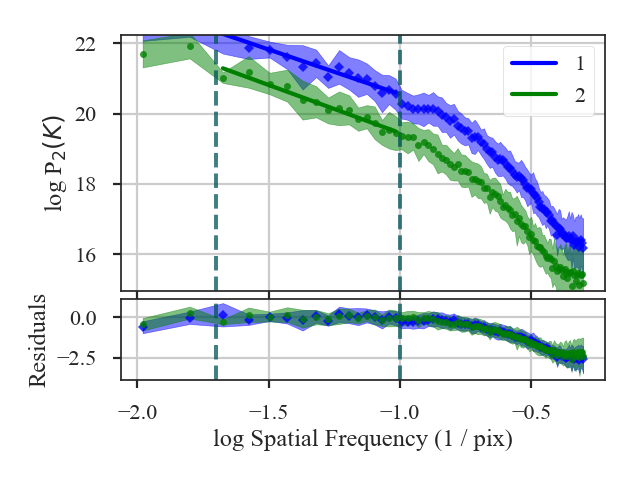
The distance is now:
>>> mvc.distance # doctest: +SKIP
0.46621655722371613
A pre-computed MVC
class can also be passed instead of giving a dataset as the input. See the distance metric introduction.